The Future of Emotion Recognition in Machine Learning and AI
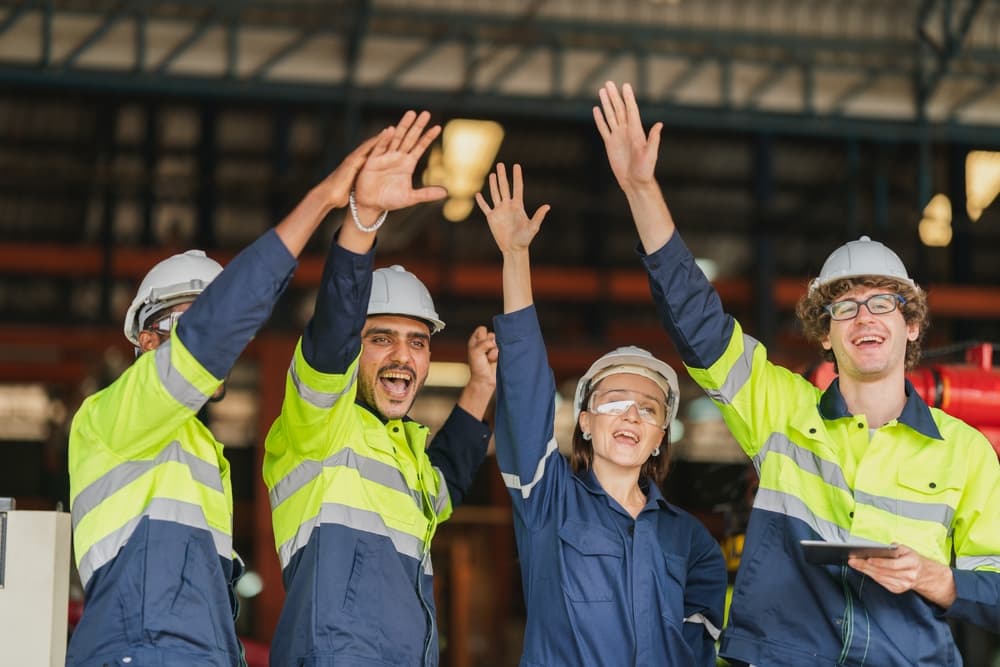
Key Takeaways
- Market Growth: The emotion detection and recognition market is projected to reach $55.86 million by 2028
- Safety Applications: Automotive AI systems can monitor driver emotions to prevent accidents
- Multiple Modalities: Beyond facial expressions, systems can detect emotions through voice, physiological signals, and even radio waves
- Technical Approach: Deep learning has become the dominant methodology for emotion recognition
- Expanding Applications: From healthcare to marketing, emotion AI is finding diverse use cases
Understanding Emotion AI
Emotion AI, also known as affective computing, represents a rapidly evolving field focused on developing systems that can detect, interpret, and respond to human emotions. As artificial intelligence continues to advance, the ability to recognize and appropriately react to emotional states has become increasingly important for creating more intuitive and responsive human-machine interactions.
Computer vision systems can analyze facial expressions to detect emotional states
At its core, emotion recognition technology aims to bridge the emotional intelligence gap between humans and machines. While humans naturally perceive emotional cues through facial expressions, voice tonality, body language, and contextual understanding, machines require sophisticated algorithms and training to recognize these same patterns.
Visual Emotion Recognition
The most developed area of emotion AI focuses on visual analysis of facial expressions. These systems typically:
- Detect faces in images or video streams
- Extract facial landmarks such as eye corners, mouth edges, and eyebrows
- Analyze micro-expressions and facial muscle movements
- Classify emotions into categories like happiness, sadness, anger, surprise, fear, and disgust
Visual emotion analysis presents significant challenges due to the "affective gap"—the disconnect between low-level pixel data and high-level emotional concepts. Despite these challenges, deep learning approaches, particularly convolutional neural networks (CNNs), have demonstrated remarkable success in bridging this gap.
Modern systems can detect subtle emotional cues even in challenging conditions such as poor lighting, partial occlusion, or when subjects are at various angles to the camera. These capabilities have opened up numerous applications across industries.
Beyond Visual: Multi-Modal Emotion Recognition
While facial expression analysis remains prominent, researchers are exploring additional modalities for emotion detection:
Voice and Speech Analysis
Systems can analyze vocal characteristics including:
- Pitch variations
- Speaking rate
- Voice intensity
- Speech pauses
- Vocal tremors
These acoustic features provide valuable emotional context, especially when combined with linguistic content analysis.
Physiological Signals
Biometric sensors can detect emotional states through:
- Heart rate variability
- Respiration patterns
- Skin conductance
- Body temperature
- Brain activity (EEG)
These signals often reveal emotions that might not be visibly expressed.
Novel Sensing Approaches
Researchers at Queen Mary University of London have demonstrated an innovative approach using radio waves—similar to radar or Wi-Fi—to detect emotions. The system:
- Transmits radio signals toward subjects
- Analyzes the reflected waves
- Detects subtle body movements associated with heart and breathing rates
- Uses these physiological patterns to infer emotional states
This technology can potentially detect emotions even when traditional visual cues are unavailable, opening new possibilities for ambient emotion sensing in smart environments.
Market Growth and Applications
According to Adroit Market Research, the global emotion detection and recognition (EDR) market was valued at USD 19.87 million in 2020 and is projected to reach USD 55.86 million by 2028. This significant growth reflects the expanding applications across multiple sectors:
Automotive Safety
One of the most promising applications is in driver monitoring systems. With distracted driving causing numerous injuries and fatalities daily, automotive manufacturers are implementing emotion AI to:
- Detect driver drowsiness and alert the driver
- Identify distraction or emotional agitation
- Monitor attention levels during semi-autonomous driving
- Adapt vehicle responses based on driver state
Companies like Affectiva have developed specialized Automotive AI that uses cameras and microphones to measure complex emotional and cognitive states in real-time. These systems help manufacturers meet increasingly stringent safety standards such as those set by the European New Car Assessment Program (Euro NCAP).
Additional Application Areas
The technology is finding diverse applications across industries:
Law Enforcement and Security
- Threat detection in public spaces
- Interview analysis
- Surveillance monitoring
Healthcare
- Mental health assessment
- Pain detection in non-verbal patients
- Therapeutic applications
Marketing and Retail
- Consumer response analysis
- Product testing
- Personalized advertising
Entertainment
- Adaptive gaming experiences
- Content recommendation
- Interactive media
Technical Approaches
The technical landscape for emotion recognition has evolved significantly:
Traditional Machine Learning
Early systems relied on:
- Handcrafted features
- Support vector machines
- Random forests
- Hidden Markov models
These approaches required extensive feature engineering and struggled with generalization.
Deep Learning
Modern systems predominantly use:
- Convolutional neural networks (CNNs)
- Recurrent neural networks (RNNs)
- Transformer models
- Multimodal fusion architectures
Deep learning approaches have significantly improved accuracy by automatically learning relevant features from large datasets.
Hybrid Approaches
State-of-the-art systems often combine:
- Multiple data modalities
- Context-aware processing
- Temporal analysis
- Cultural and individual calibration
These hybrid approaches help address the complex, subjective nature of emotion.
Challenges and Considerations
Despite rapid progress, emotion recognition technology faces several challenges:
Technical Limitations
- Accuracy in uncontrolled environments
- Cultural and individual differences in emotional expression
- Distinguishing between genuine and posed emotions
- Real-time processing requirements
Ethical Considerations
- Privacy concerns regarding constant emotional monitoring
- Potential for manipulation or exploitation
- Bias in training data and algorithms
- Consent for emotional data collection
Regulatory Landscape
- Emerging regulations on biometric data
- Industry-specific compliance requirements
- International variations in permitted applications
Future Directions
The future of emotion recognition in AI is likely to develop along several trajectories:
Enhanced Contextual Understanding
Systems will increasingly incorporate situational context to improve accuracy and relevance of emotion detection.
Personalized Calibration
Future systems will adapt to individual emotional expression patterns rather than relying solely on universal models.
Multimodal Integration
The most effective systems will seamlessly combine multiple sensing modalities to create more robust emotion recognition.
Emotion-Responsive Interfaces
Human-computer interfaces will evolve to dynamically respond to user emotional states, creating more natural interactions.
Preventive Applications
Proactive systems will identify emotional patterns that precede negative outcomes and intervene appropriately.
Conclusion
Emotion recognition represents a crucial frontier in artificial intelligence, potentially transforming how machines understand and interact with humans. From automotive safety applications that prevent accidents to healthcare systems that better assess patient needs, the technology offers significant benefits across numerous domains.
As the field continues to mature, balancing technological advancement with ethical considerations will be essential. The most successful implementations will likely be those that enhance human capabilities and wellbeing while respecting privacy and individual autonomy.
For organizations exploring emotion AI applications, understanding both the technical capabilities and the responsible implementation frameworks will be key to creating systems that genuinely improve human-machine interaction.
This article provides a historical perspective on emotion recognition technology. While Visionify now specializes in computer vision solutions for various industries, we recognize the continuing importance of affective computing in creating more intuitive and responsive AI systems.
Want to learn more?
Discover how our Vision AI safety solutions can transform your workplace safety.
Schedule a DemoSchedule a Meeting
Book a personalized demo with our product specialists to see how our AI safety solutions can work for your business.
Choose a convenient time
Select from available slots in your timezone
30-minute consultation
Brief but comprehensive overview of our solutions
Meet our product experts
Get answers to your specific questions
Related Articles
Subscribe to our newsletter
Get the latest safety insights and updates delivered to your inbox.