Opportunities of Artificial Intelligence in Redefining Food Inspection Industry
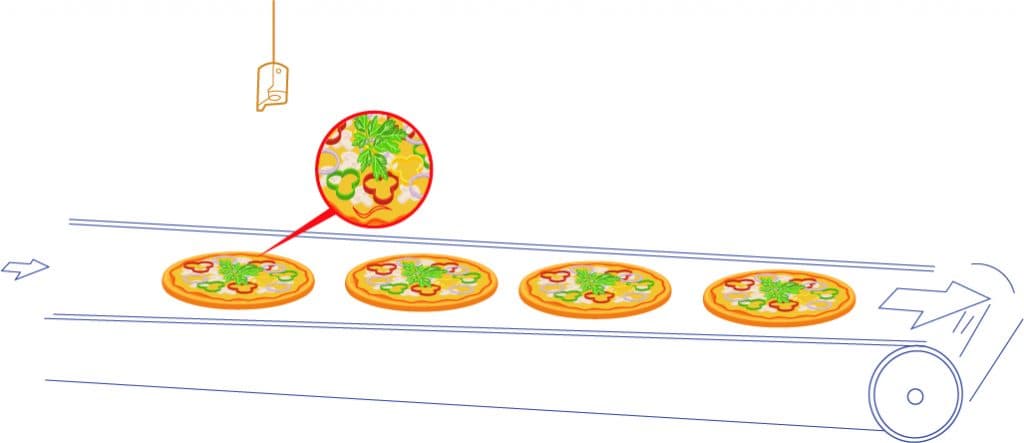
Key Takeaways
- Economic Impact: Foodborne illnesses cost approximately $110 billion annually worldwide
- Early Detection: AI systems can predict food recalls with up to 74% accuracy using consumer reviews
- Contamination Prevention: Computer vision can detect foreign objects and debris on production lines
- Shelf-Life Prediction: AI algorithms can forecast food decay based on environmental monitoring
- Waste Reduction: Smart monitoring helps reduce the 1.3 billion tonnes of food wasted annually
The Food Safety Challenge
Food safety represents one of the most critical public health challenges globally. According to the World Bank, foodborne illnesses cost approximately $110 billion per year in lost productivity and medical expenses. Traditional inspection methods, while valuable, face significant limitations in scale, consistency, and speed—creating opportunities for technological innovation.
AI-powered computer vision systems can detect contamination and foreign objects that might be missed by human inspectors
The global food safety testing market was valued at over $18 billion in 2019 according to Adroit Market Research, reflecting the significant investment in ensuring food products meet safety standards. However, traditional testing methods often involve time-consuming laboratory analysis and sampling approaches that cannot provide 100% inspection coverage.
AI Applications Transforming Food Inspection
Artificial intelligence is revolutionizing food safety through several key applications:
1. Foreign Object Detection
One of the most immediate applications of AI in food safety is the detection of foreign objects and contaminants on production lines. Computer vision systems equipped with specialized cameras and deep learning algorithms can:
- Identify foreign materials including plastic, metal, glass, and organic contaminants
- Detect objects that are difficult for humans to see consistently
- Operate continuously without fatigue or attention lapses
- Process thousands of items per minute with consistent accuracy
- Integrate with rejection systems to remove contaminated products
These systems typically use a combination of visible light, infrared, and hyperspectral imaging to detect contaminants even when they're similar in color to the food product itself. The AI algorithms are trained on thousands of examples to recognize normal products and flag anomalies.
2. Predictive Analytics for Food Recalls
Researchers have developed AI systems that can predict potential food safety issues before they result in widespread illness or recalls:
- The FDA has explored BERT (Bidirectional Encoder Representations from Transformers) algorithms to analyze consumer reviews
- These systems can identify patterns in consumer complaints that correlate with unsafe products
- Early research shows up to 74% accuracy in predicting recalls based on review analysis
- This approach could significantly accelerate the recall process, potentially reducing illness cases
By analyzing thousands of reviews and correlating them with previous recalls, these systems identify key phrases and patterns that indicate potential safety issues, allowing for earlier intervention.
3. Decay Prediction and Shelf-Life Management
AI systems are also being developed to predict food spoilage before it occurs:
- Sensor networks monitor temperature, humidity, and gas levels in storage environments
- Machine learning algorithms analyze this data to predict remaining shelf life
- Systems can alert managers when products are approaching critical points
- This technology helps reduce the estimated 1.3 billion tonnes of food wasted annually
These predictive systems are particularly valuable for perishable products and can help optimize inventory management to reduce waste while ensuring food safety.
4. Automated Quality Grading
Beyond safety concerns, AI systems can assess food quality attributes:
- Evaluating fruit ripeness and detecting bruising
- Grading meat products for quality and consistency
- Assessing grain quality and detecting mold or mycotoxins
- Verifying product specifications for size, shape, and appearance
These applications help ensure consistent quality while freeing human inspectors to focus on more complex assessment tasks.
Implementation Considerations
Organizations implementing AI for food inspection should consider several key factors:
1. System Integration
- Production Line Compatibility: Ensuring systems can operate at production speeds
- Rejection Mechanisms: Implementing appropriate methods to remove flagged products
- Data Management: Storing inspection data for traceability and continuous improvement
- Existing Systems: Integration with quality management and production systems
2. Training Requirements
- Algorithm Development: Creating models specific to particular food products
- Continuous Learning: Updating systems as new products or contaminants emerge
- Staff Training: Preparing quality teams to work with AI-augmented systems
- Validation Protocols: Establishing procedures to verify system performance
3. Regulatory Compliance
- Documentation: Ensuring systems meet regulatory requirements for food safety
- Validation: Demonstrating system effectiveness to regulatory authorities
- Audit Support: Using AI data to support food safety audits
- Standards Alignment: Ensuring compatibility with HACCP, GFSI, and other frameworks
Return on Investment
The business case for AI in food inspection typically includes several components:
- Recall Prevention: Avoiding the substantial costs of product recalls
- Brand Protection: Preventing reputation damage from safety incidents
- Labor Efficiency: Optimizing the use of quality personnel
- Waste Reduction: Minimizing discarded product through better prediction
- Throughput Improvement: Maintaining production speeds with confidence
For most implementations, these benefits combine to deliver positive ROI within 12-24 months.
Future Directions
As AI technology continues to evolve, several emerging trends will further transform food inspection:
- Portable Devices: Handheld sensors with AI capabilities for field testing
- Blockchain Integration: Connecting inspection data with supply chain traceability
- Consumer Applications: Empowering consumers with food safety verification tools
- Predictive Maintenance: Anticipating equipment issues that could affect food safety
- Autonomous Systems: Fully automated inspection and sorting systems
Conclusion
Artificial intelligence represents a transformative opportunity for the food inspection industry, offering unprecedented capabilities for contamination detection, quality assessment, and predictive analytics. As these technologies mature and become more accessible, they will play an increasingly important role in ensuring food safety while improving operational efficiency.
For food manufacturers, processors, and regulatory agencies, AI-powered inspection systems provide a powerful tool for addressing the persistent challenges of foodborne illness and product quality. By embracing these technologies, the industry can move toward more proactive, comprehensive, and effective food safety management.
This article provides a historical perspective on AI in food inspection. While Visionify now specializes in computer vision solutions for various industries, we recognize the continuing importance of food safety applications for artificial intelligence and computer vision technology.
Want to learn more?
Discover how our Vision AI safety solutions can transform your workplace safety.
Schedule a DemoSchedule a Meeting
Book a personalized demo with our product specialists to see how our AI safety solutions can work for your business.
Choose a convenient time
Select from available slots in your timezone
30-minute consultation
Brief but comprehensive overview of our solutions
Meet our product experts
Get answers to your specific questions
Related Articles
Subscribe to our newsletter
Get the latest safety insights and updates delivered to your inbox.