How does Real-time Fall Detection using Pose Estimation work?
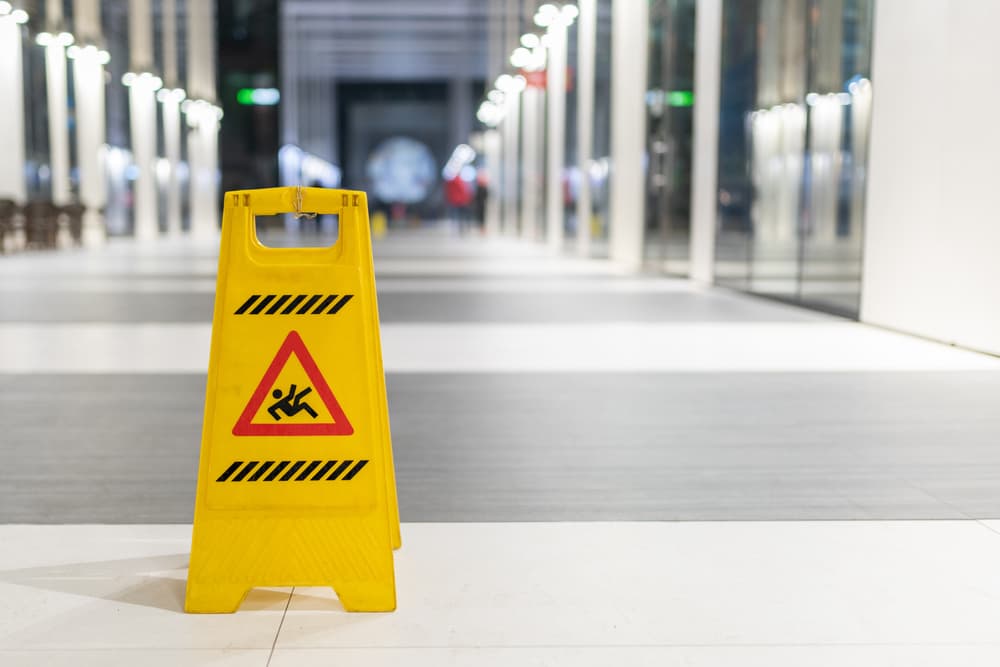
Key Takeaways
- Life-Saving Technology: Fall detection systems can automatically alert caregivers when falls occur
- Pose Estimation: AI identifies key body points to track human movement and detect abnormal patterns
- Real-Time Processing: Modern systems can analyze video feeds at 6+ frames per second
- High Accuracy: Advanced algorithms achieve up to 90% accuracy in fall detection
- Multiple Applications: Benefits elderly care, hospitals, rehabilitation centers, and independent living
Understanding Pose Estimation
Pose estimation is a computer vision technique that locates human body joints—such as elbows, wrists, knees, and ankles—in images or video frames. These joints, known as keypoints, are identified and connected to create a skeletal representation of the human body.
This technology forms the foundation of fall detection systems by enabling computers to understand human movement patterns and identify abnormal motions that indicate a fall has occurred.
The Fall Detection Process
Real-time fall detection systems using pose estimation typically follow a multi-stage process:
1. Video Input Processing
The system begins by capturing video from cameras installed in the monitoring environment. Multiple camera streams can be processed simultaneously using parallel computing techniques to cover larger areas or different rooms.
2. Person Detection and Pose Estimation
For each video frame, the system:
- Identifies human figures in the scene
- Locates key body points for each person
- Creates a skeletal model by connecting these points
Modern systems use deep learning models like OpenPifPaf that can accurately detect human poses even in challenging conditions such as poor lighting or partial occlusion.
3. Person Tracking
To monitor individuals continuously across frames, the system must track each person's identity. This is accomplished through:
- Computing the centroid (center point) of each detected person
- Assigning unique IDs to each centroid
- Calculating the distance between centroids in consecutive frames
- Maintaining ID consistency by matching the closest centroids between frames
This tracking allows the system to follow individuals even as they move throughout the monitored space.
4. Fall Detection Algorithm
The core of the system analyzes the tracked pose data to identify falls using several approaches:
Dimensional Analysis
- Monitoring changes in the height-to-width ratio of a person's bounding box
- A sudden change from a vertical orientation (standing) to horizontal (lying) may indicate a fall
Key Point Relationship Analysis
- Tracking the relative positions of critical points like the head, neck, and ankles
- Analyzing the velocity and acceleration of these points
- Identifying the characteristic rapid downward movement followed by sudden stillness that typifies a fall
Temporal Pattern Recognition
- Examining sequences of poses over time rather than single frames
- Distinguishing between normal activities (sitting, bending) and actual falls
5. Alert Generation
When a fall is detected, the system triggers an alert that can be:
- Sent to caregivers' mobile devices
- Transmitted to a monitoring center
- Connected to emergency services
- Logged for later review and analysis
Technical Implementation
Effective fall detection systems balance accuracy with processing speed to enable real-time monitoring:
Pre-trained Models
Most implementations use pre-trained deep learning models that have been specifically optimized for human pose estimation. These models have already learned to recognize human figures and joint positions from thousands or millions of training images.
Multi-stream Processing
To handle multiple camera feeds simultaneously, systems employ parallel processing techniques:
- Each video stream is processed by a separate computing thread
- Results are aggregated in a central monitoring system
- This approach maximizes the use of available computing resources
Performance Considerations
Real-time fall detection requires efficient processing:
- Testing on NVIDIA Quadro GV100s has demonstrated processing speeds of approximately 6 frames per second
- This rate is sufficient for effective fall detection while maintaining reasonable hardware requirements
- Edge computing devices can be deployed to reduce latency and bandwidth usage
Accuracy and Validation
The effectiveness of fall detection systems is measured through rigorous testing:
- Using standardized datasets like the UR Fall Detection Dataset
- Evaluating detection rates across various fall scenarios
- Measuring false positive and false negative rates
Current systems have achieved detection rates of approximately 83% with F1 scores (a measure of accuracy) around 90%, demonstrating their potential for real-world applications.
Applications
Fall detection technology has numerous applications:
Elderly Care
- Monitoring seniors living independently
- Providing peace of mind to family members
- Enabling faster emergency response
Healthcare Settings
- Enhancing patient safety in hospitals
- Monitoring rehabilitation progress
- Reducing staff burden in care facilities
Special Populations
- Monitoring individuals with mobility issues
- Supporting people with neurological conditions that increase fall risk
- Protecting workers in high-risk environments
Challenges and Future Directions
While current fall detection systems show promise, several challenges remain:
- Occlusion Handling: Improving detection when parts of the body are hidden
- Privacy Concerns: Balancing monitoring with personal privacy
- False Alarm Reduction: Distinguishing falls from similar movements
- Integration: Connecting with existing healthcare and emergency systems
Future developments will likely focus on:
- More sophisticated AI models with higher accuracy
- Lightweight implementations for edge devices
- Multi-modal systems combining video with wearable sensors
- Predictive capabilities to identify fall risk before incidents occur
Conclusion
Real-time fall detection using pose estimation represents a significant advancement in safety monitoring technology. By leveraging computer vision and artificial intelligence, these systems can identify falls as they happen and initiate immediate response, potentially saving lives and reducing the severity of injuries.
As the technology continues to mature, we can expect more widespread adoption in healthcare facilities, assisted living environments, and private homes, providing greater independence for vulnerable populations while ensuring their safety.
This article provides a historical perspective on fall detection technology. While Visionify now specializes in computer vision solutions for various industries, we recognize the continuing importance of pose estimation technology in healthcare and safety applications.
Frequently Asked Questions
Find answers to common questions about this topic
Want to learn more?
Discover how our Vision AI safety solutions can transform your workplace safety.
Schedule a DemoSchedule a Meeting
Book a personalized demo with our product specialists to see how our AI safety solutions can work for your business.
Choose a convenient time
Select from available slots in your timezone
30-minute consultation
Brief but comprehensive overview of our solutions
Meet our product experts
Get answers to your specific questions
Subscribe to our newsletter
Get the latest safety insights and updates delivered to your inbox.